On November 11, 2024, the Department of Information Technology in the College of Engineering and Computer Science held a national workshop entitled: Machine Learning based Feature Extraction Techniques for Epileptic Seizure Detection using EEG Bio-Signals which was presented by Asst. Prof. Ashish Sharma
Workshop Objectives:
- Introduction to EEG Signals: To provide a foundational understanding of Electroencephalography (EEG), its significance, and its application in detecting brain activity.
- Exploration of Machine Learning Techniques: To delve into machine learning-based feature extraction techniques used for analyzing EEG bio-signals for epileptic seizure detection.
- Data Processing and Methodology: To present a step-by-step methodology for processing raw EEG data into a usable format for analysis.
- Feature Extraction Techniques: To discuss various techniques like Correlation-Based Feature Selection (CBFS), Information Gain Feature Selection (IGFS), Recursive Feature Elimination (RFE), and others for identifying relevant features.
- Visualization and Interpretation: To highlight critical EEG channels contributing to seizure prediction through visualization and scoring methods.
Workshop Outcomes:
- Identification of Relevant Features: Participants learn to identify significant EEG channels (e.g., T7-P7, P7-O1) for accurate seizure prediction, based on the results of feature selection techniques.
- Practical Understanding of EEG Data: Through various feature selection methods, participants understand how to refine and preprocess EEG data for improved model performance in seizure detection.
- Modeling for Seizure Detection: Understanding the importance of different EEG channels and their role in building accurate prediction models for epileptic seizures.
- Knowledge of Feature Importance: Participants will gain insights into the role of feature importance scores, especially channels like T7-P7 and FT9-FT10, in the success of prediction models.
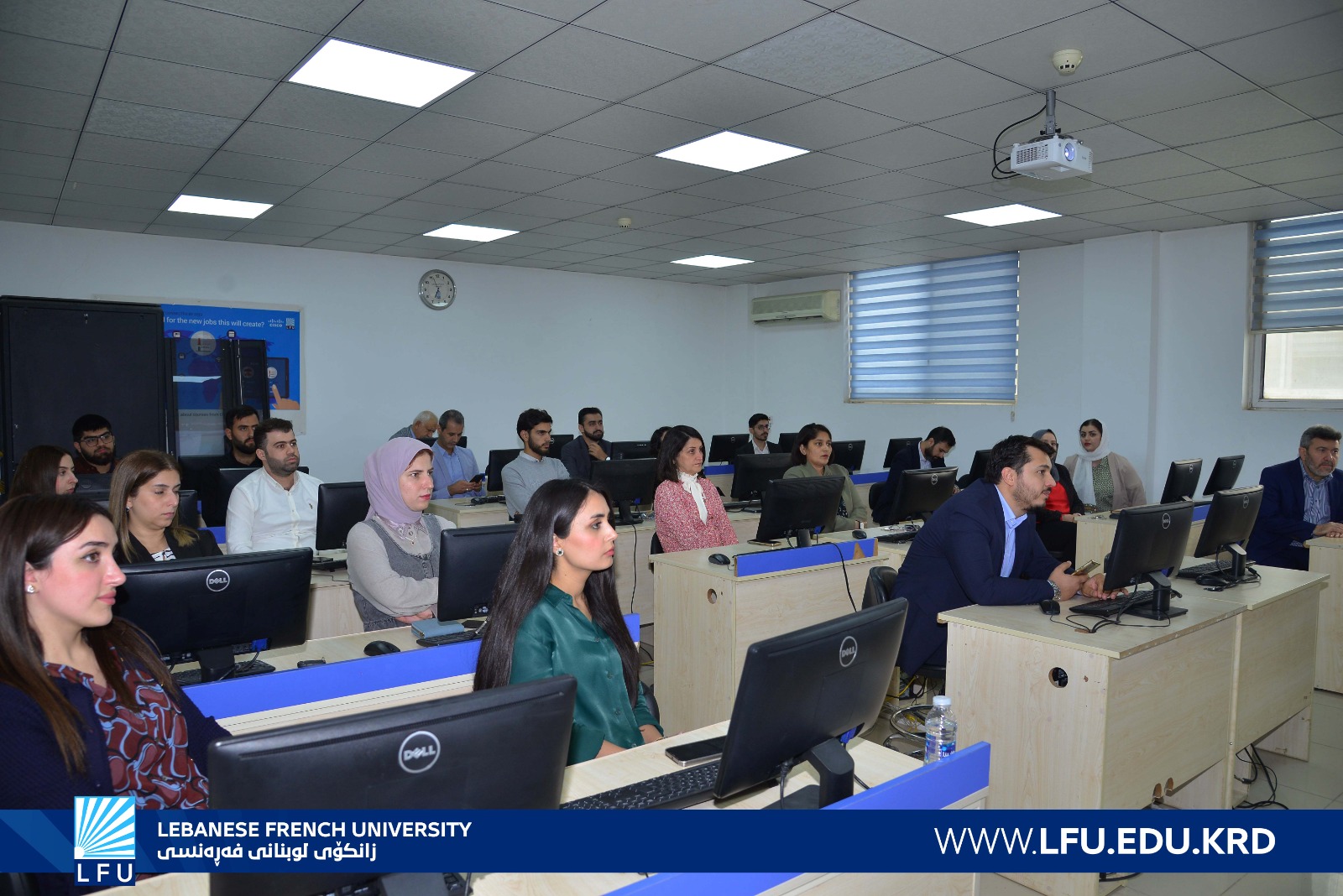
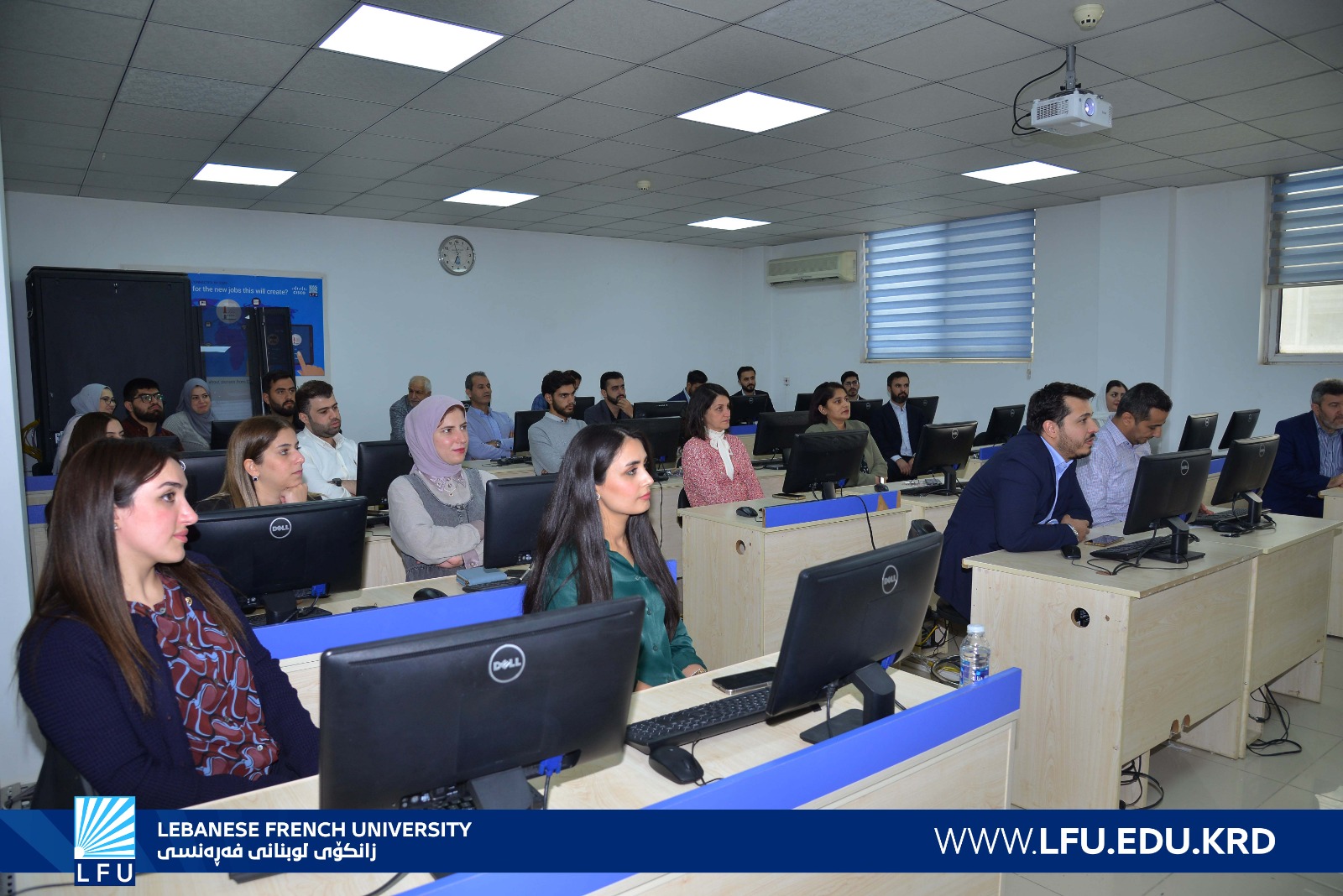
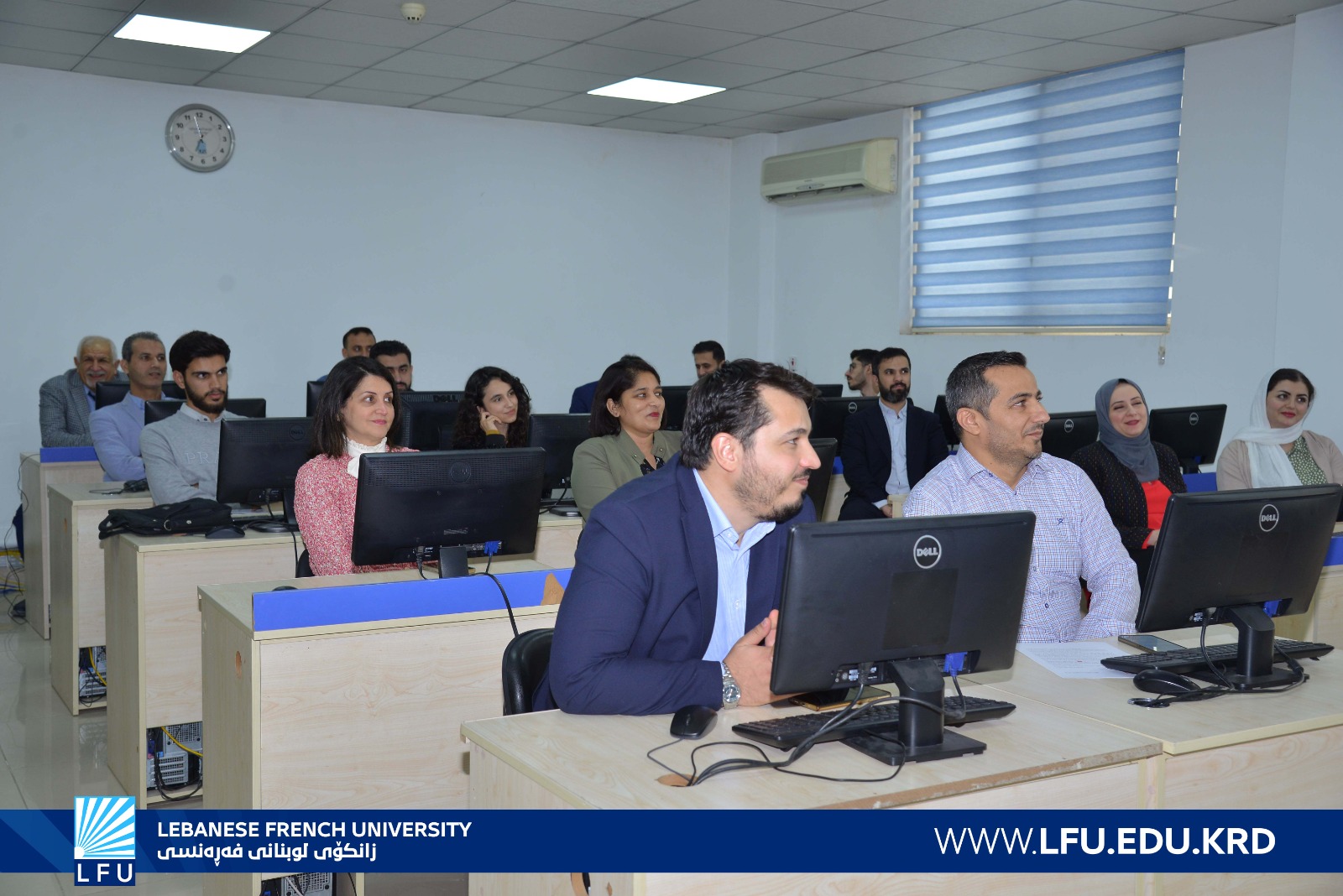
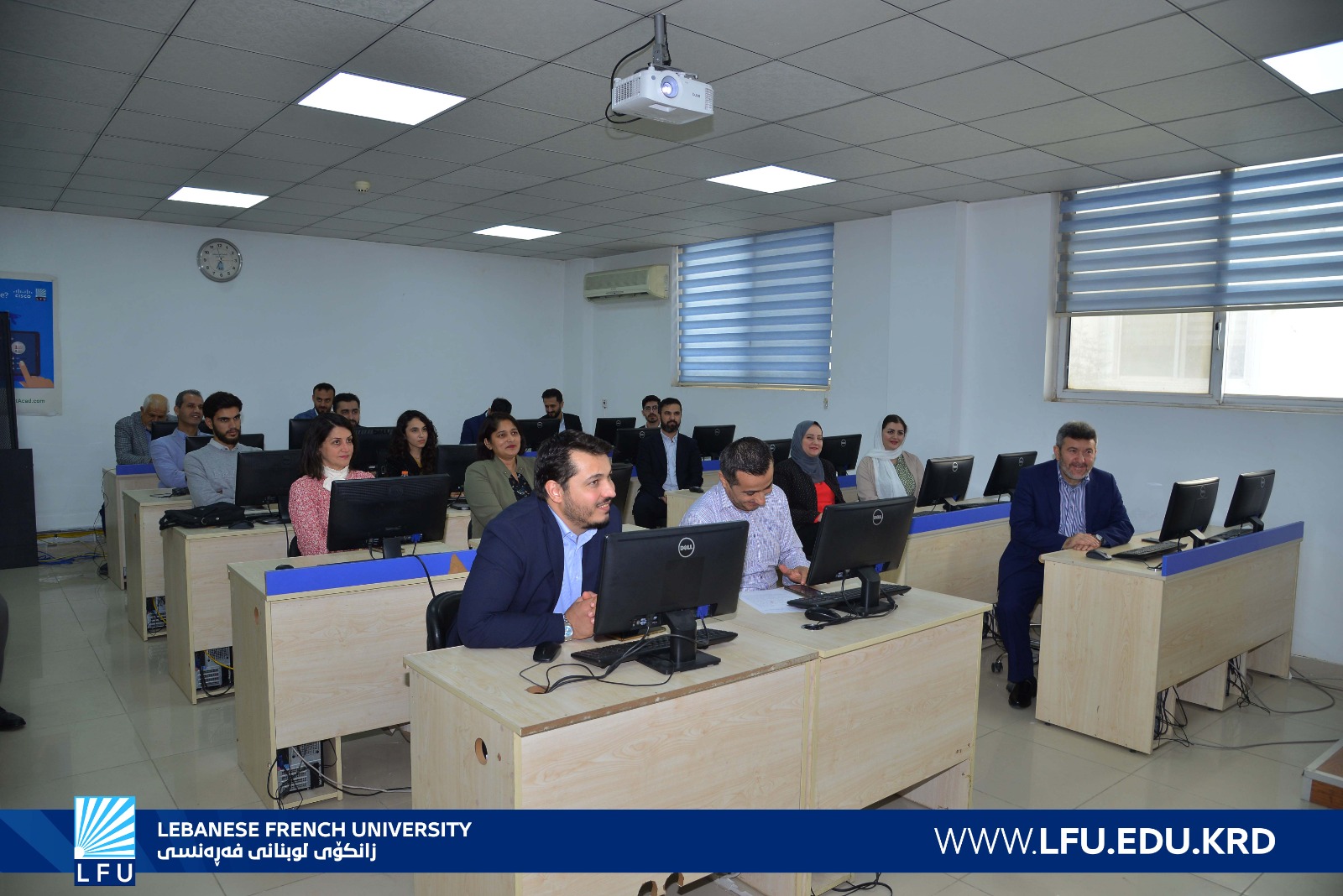
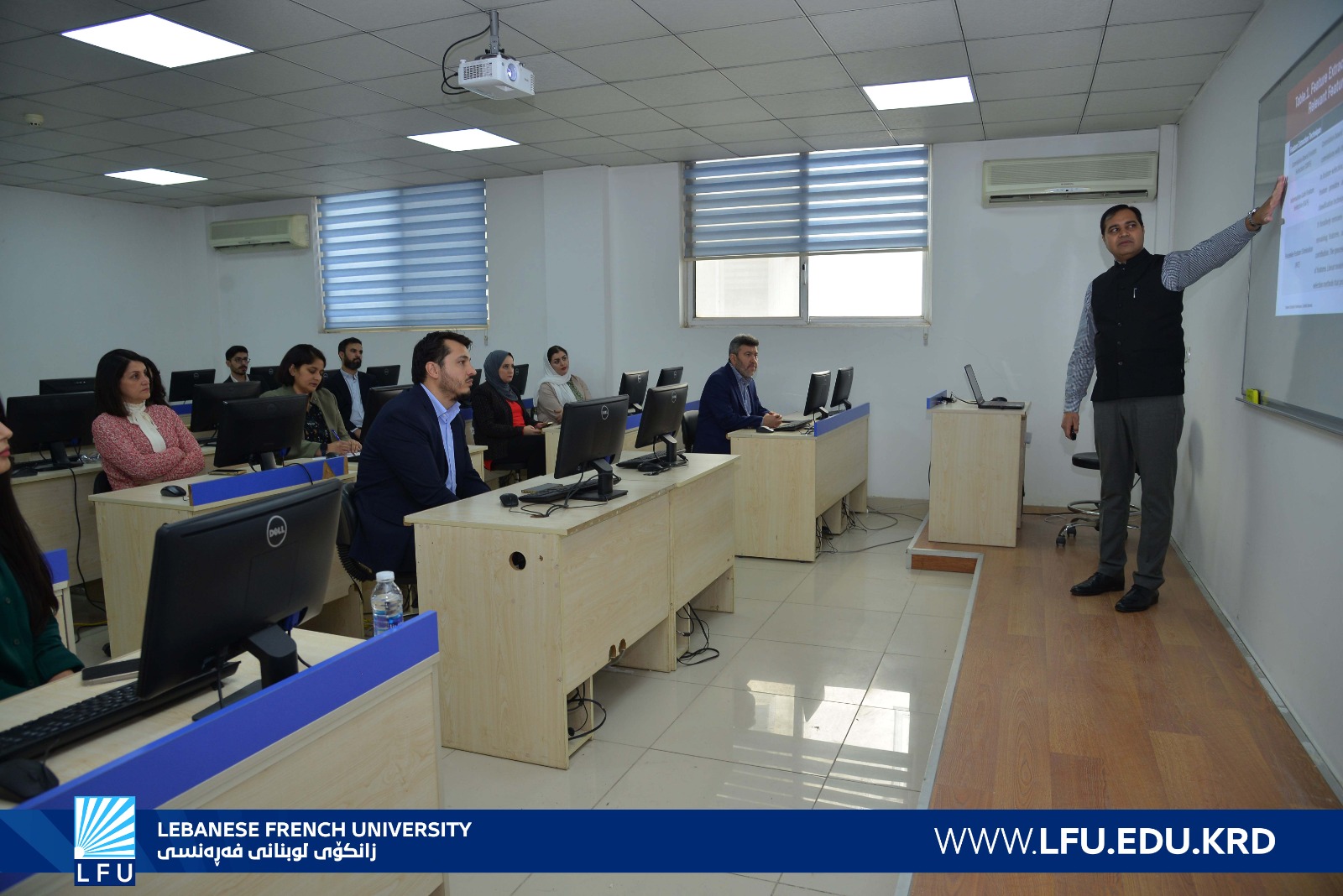
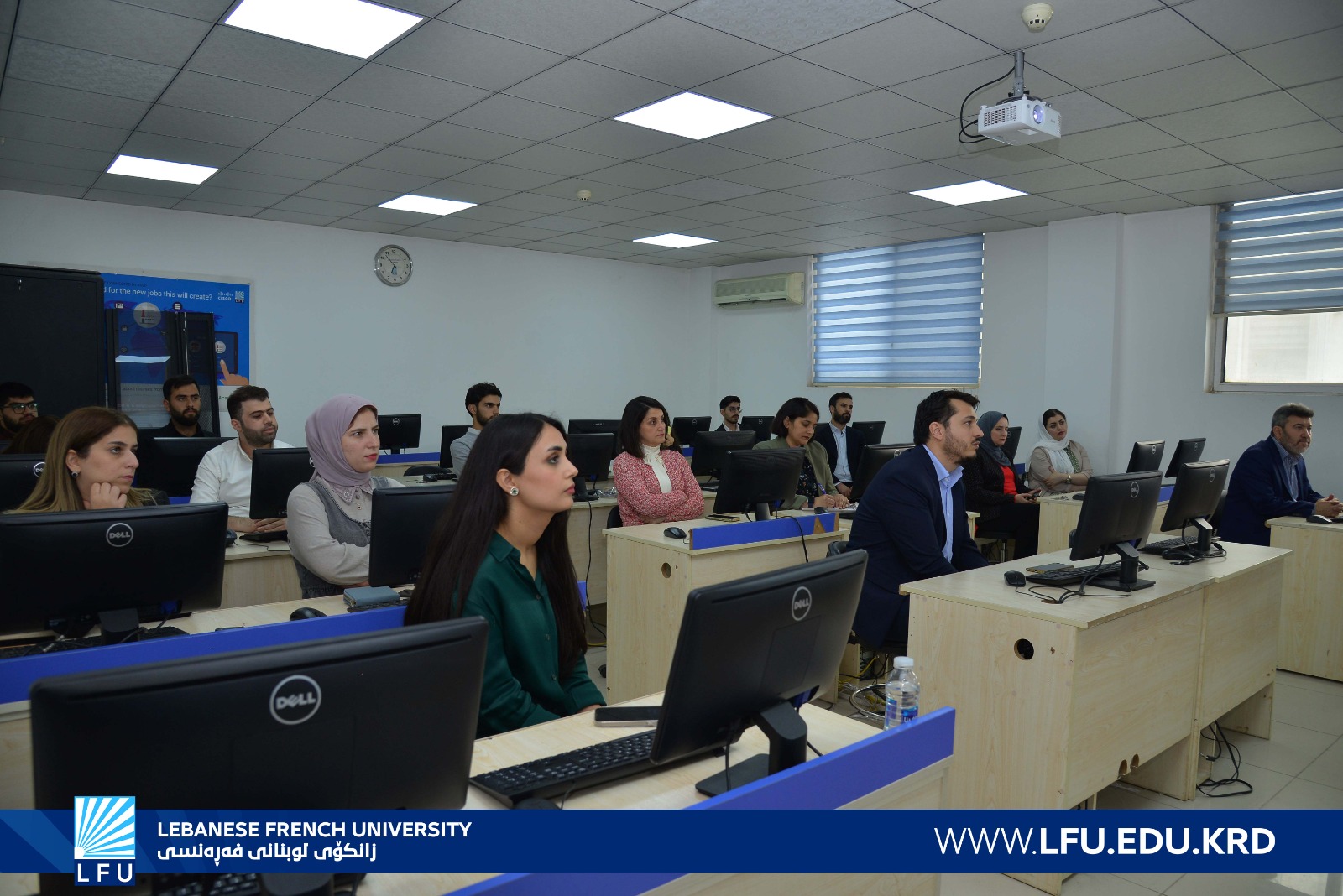
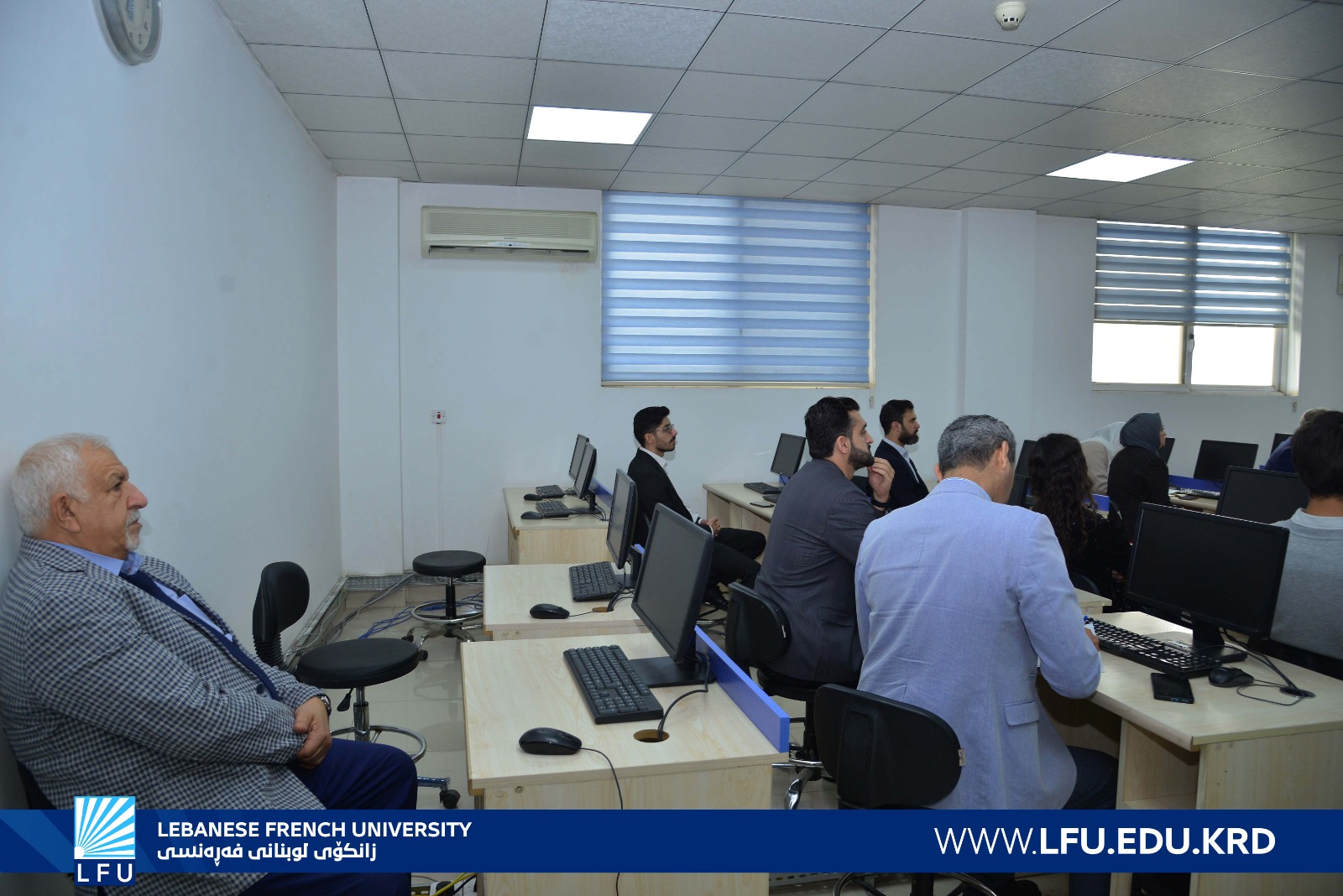
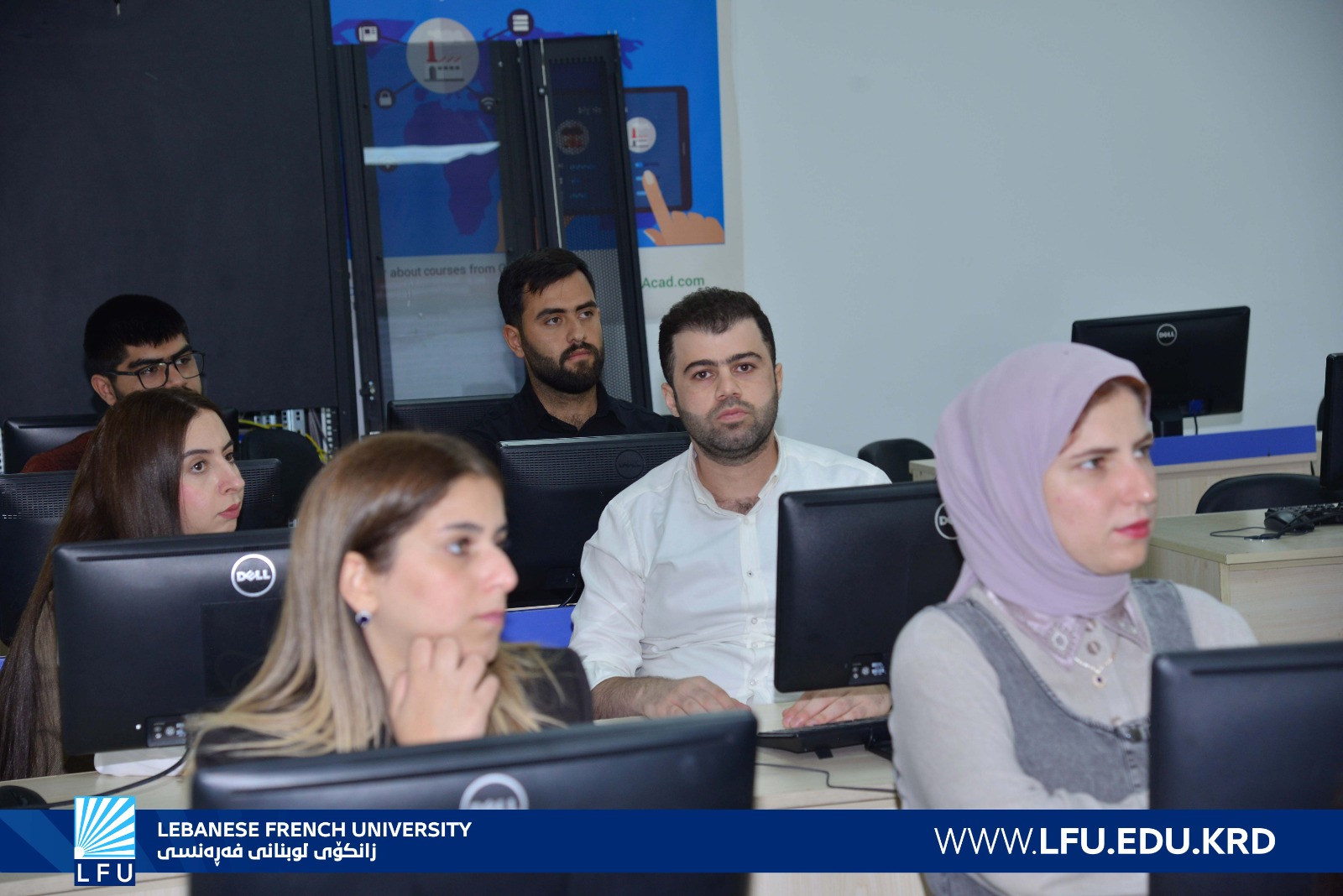
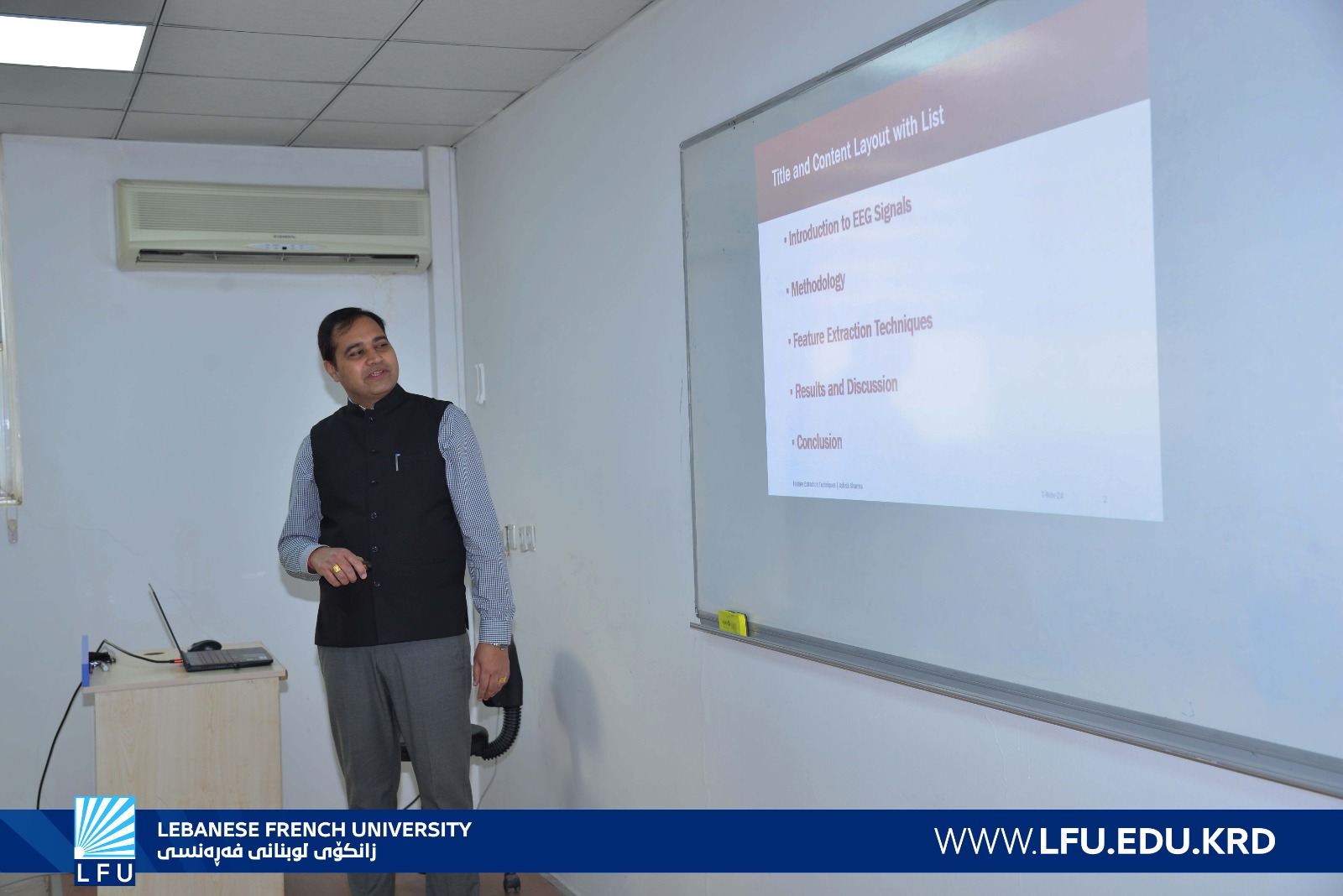
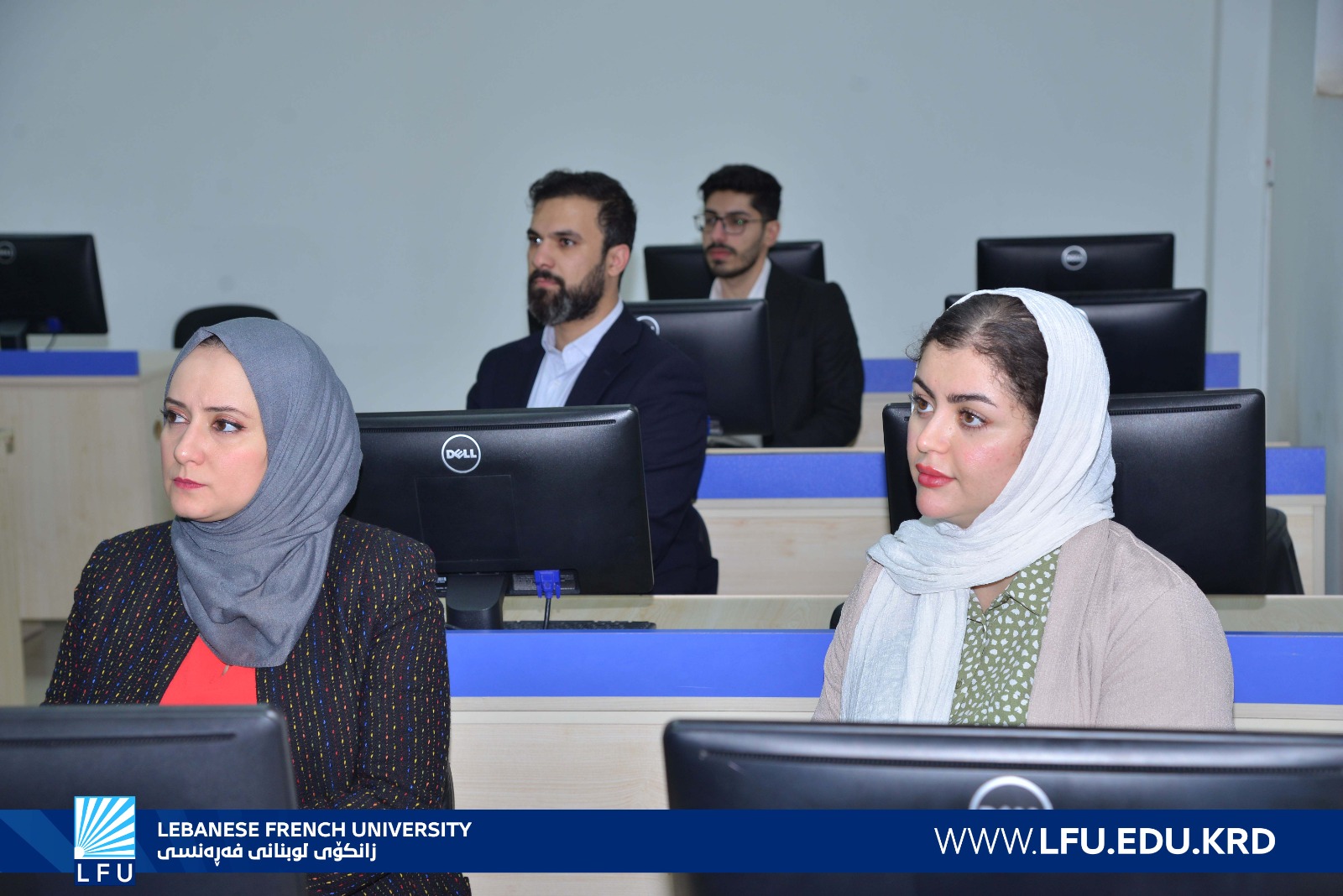
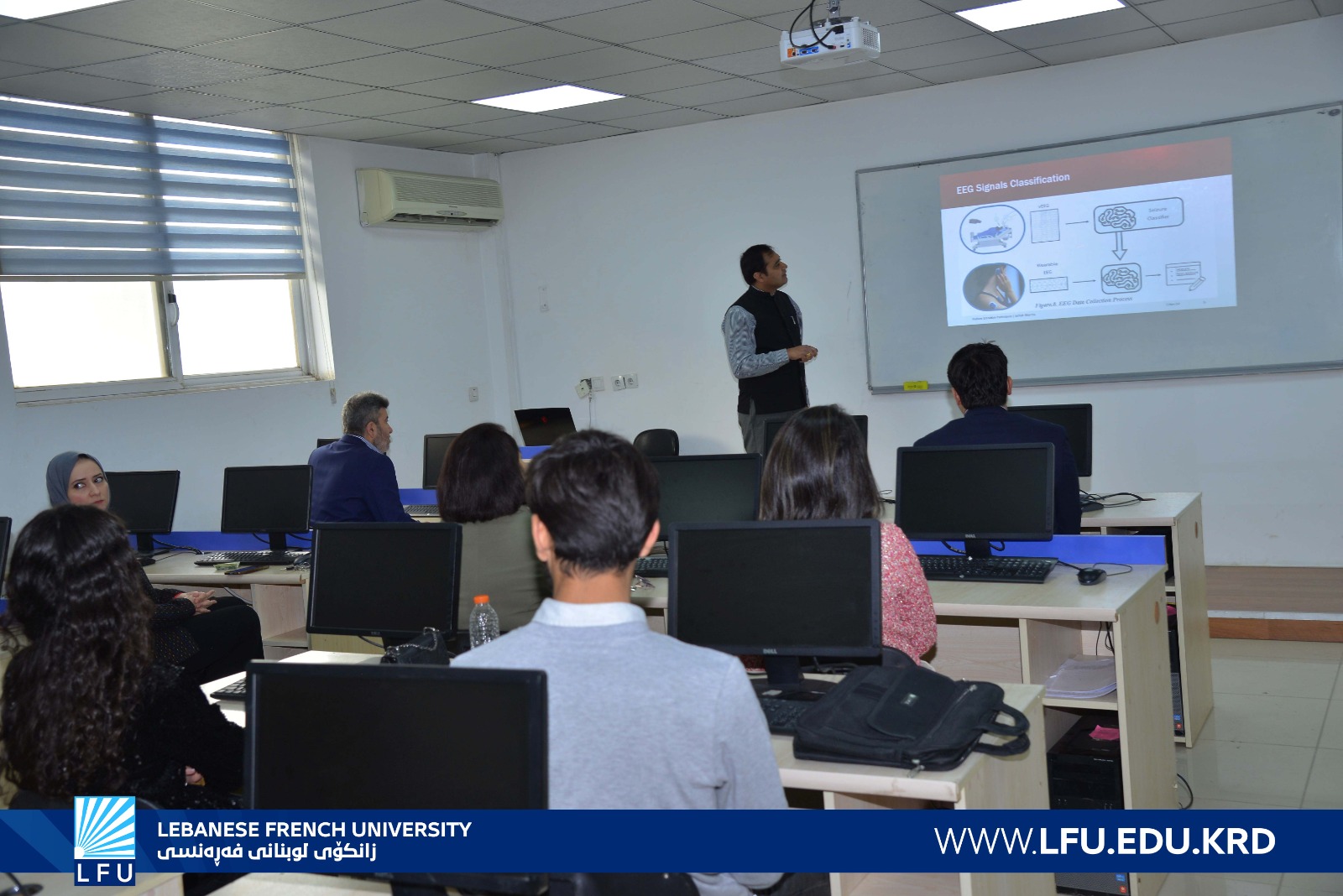
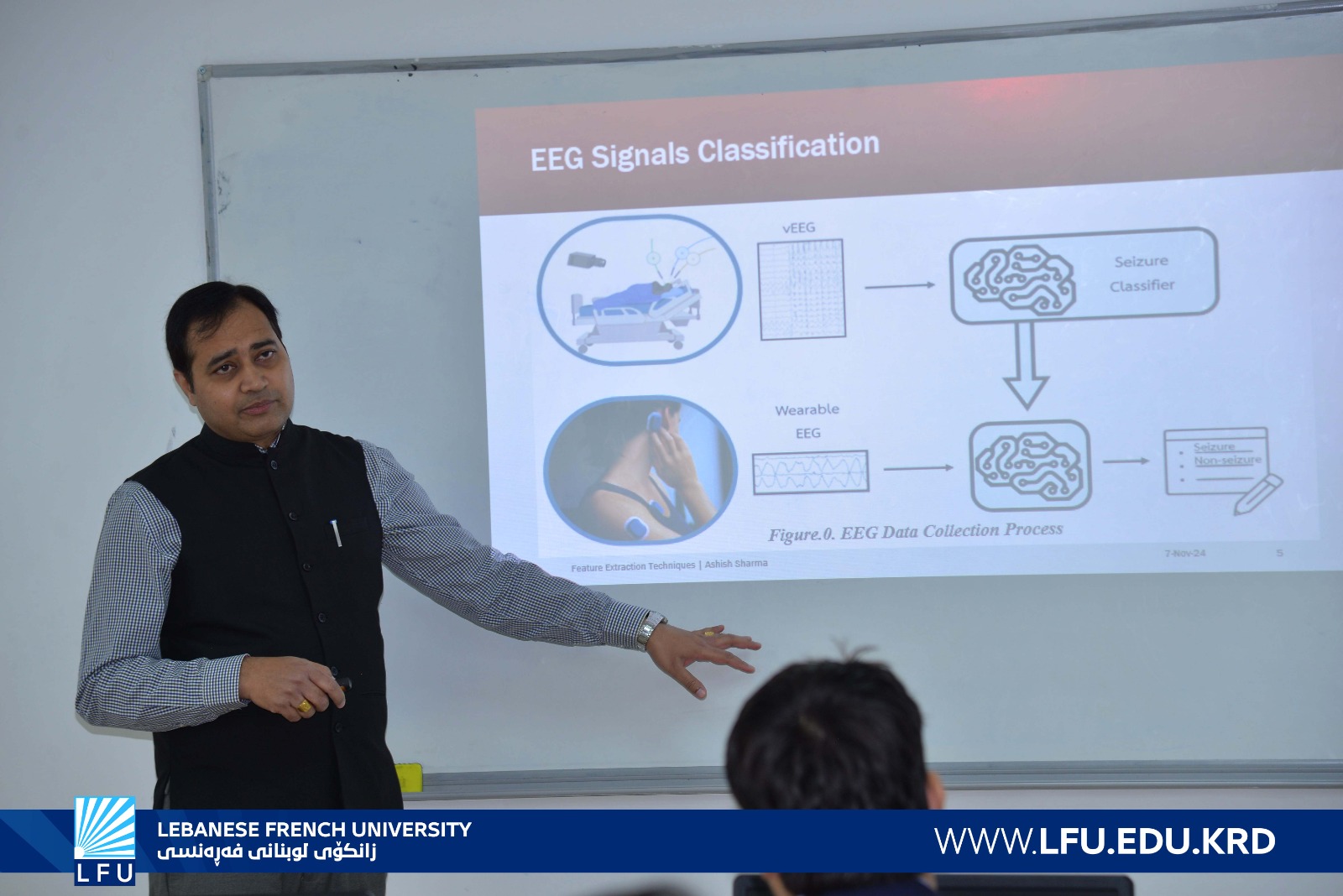
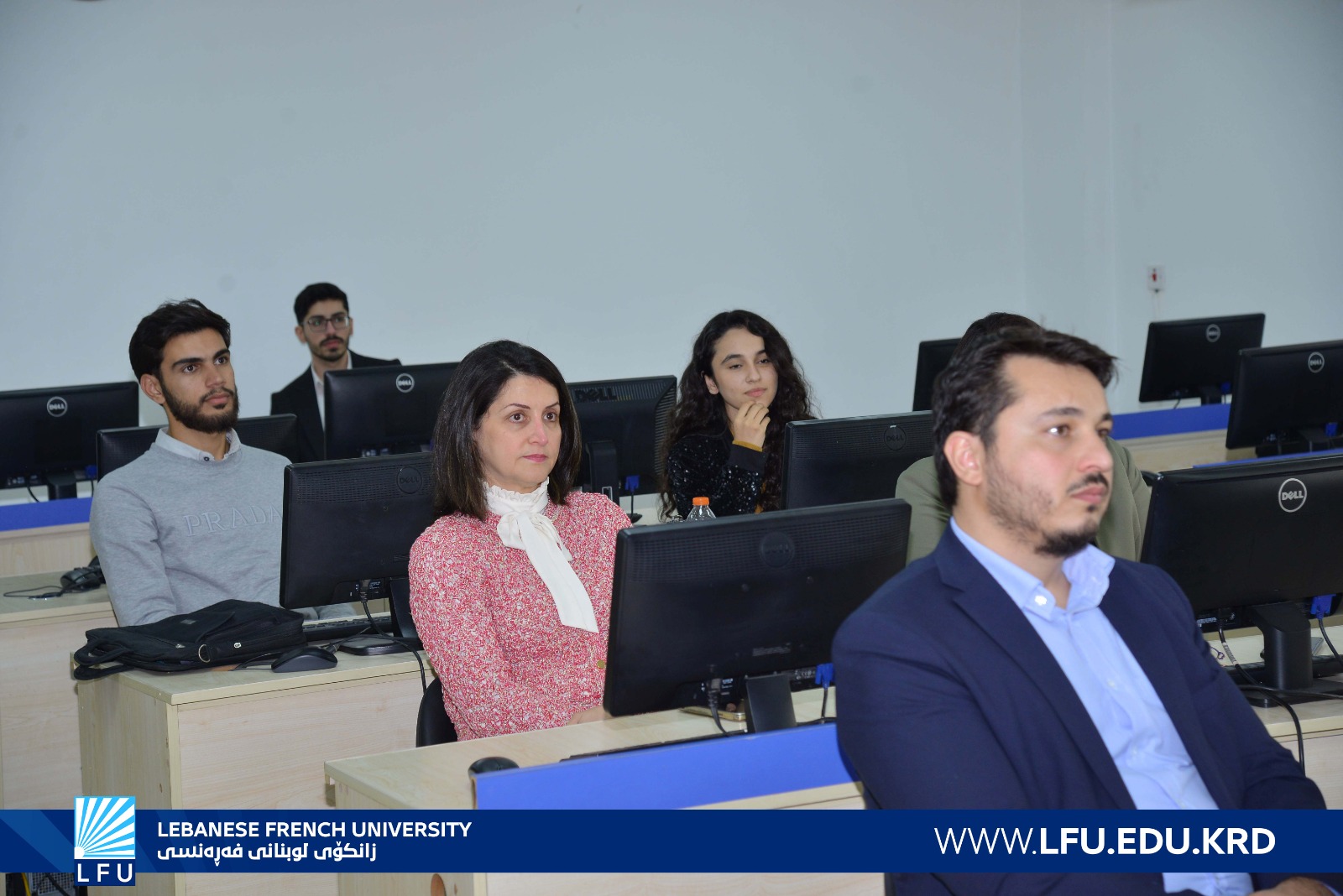
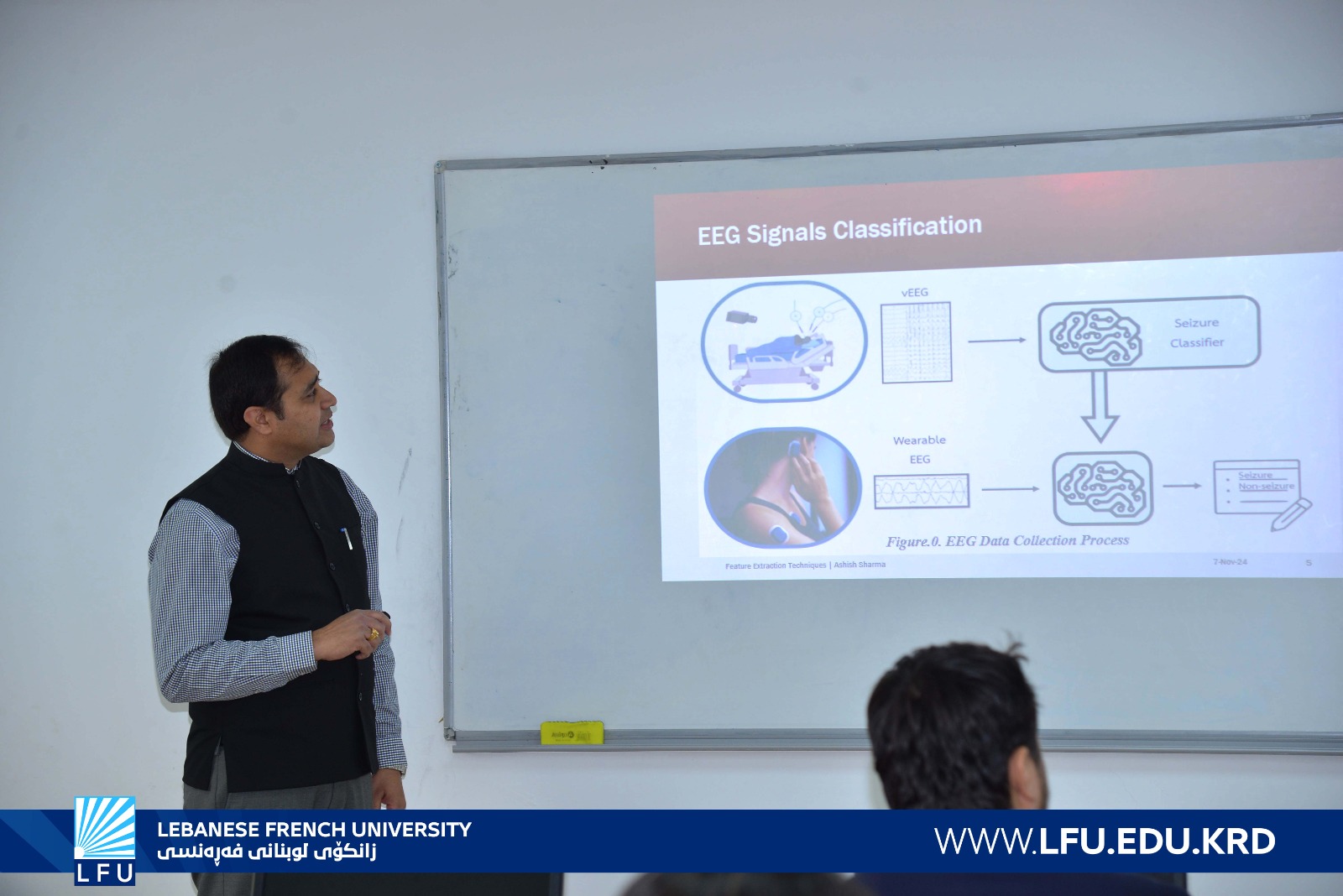
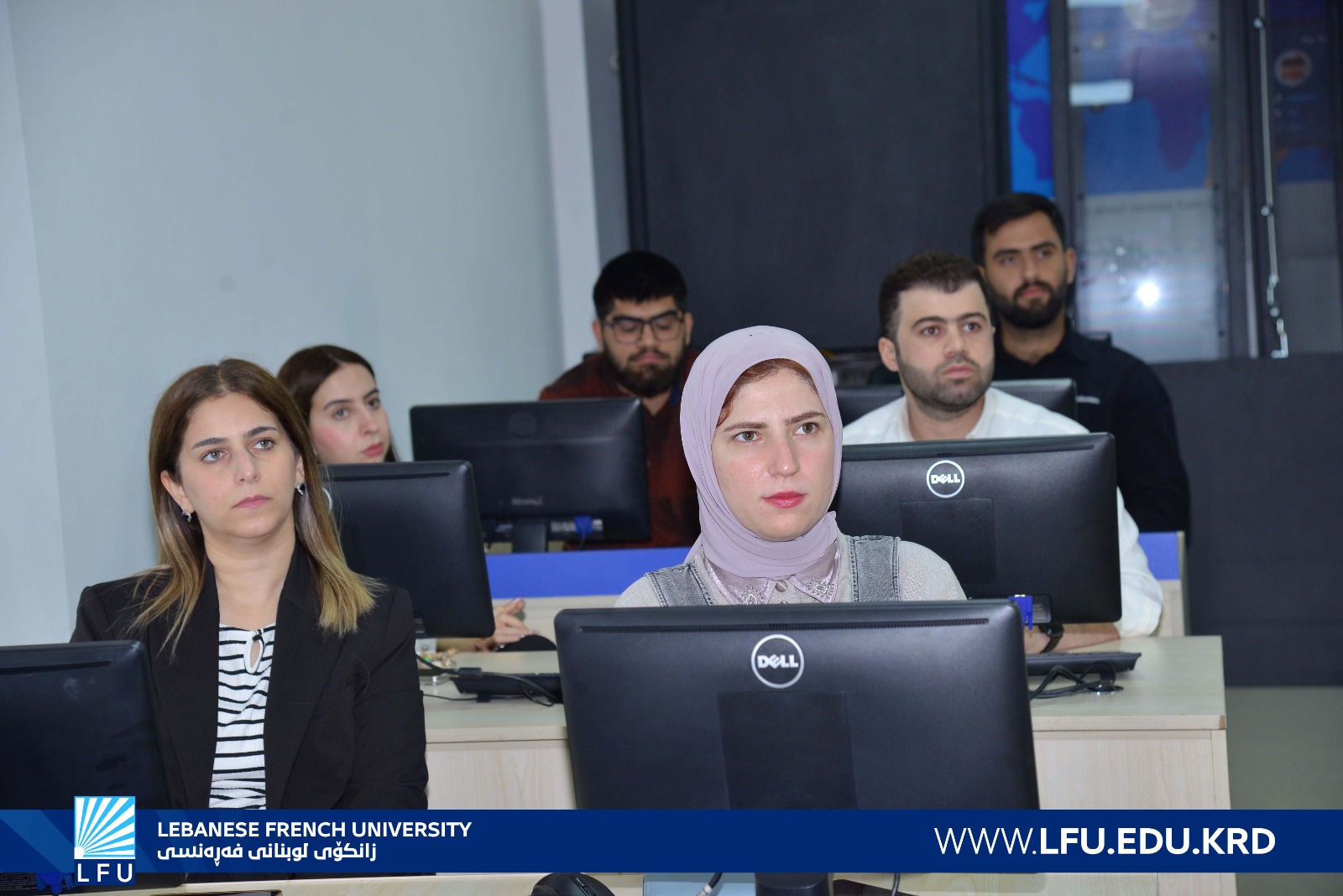
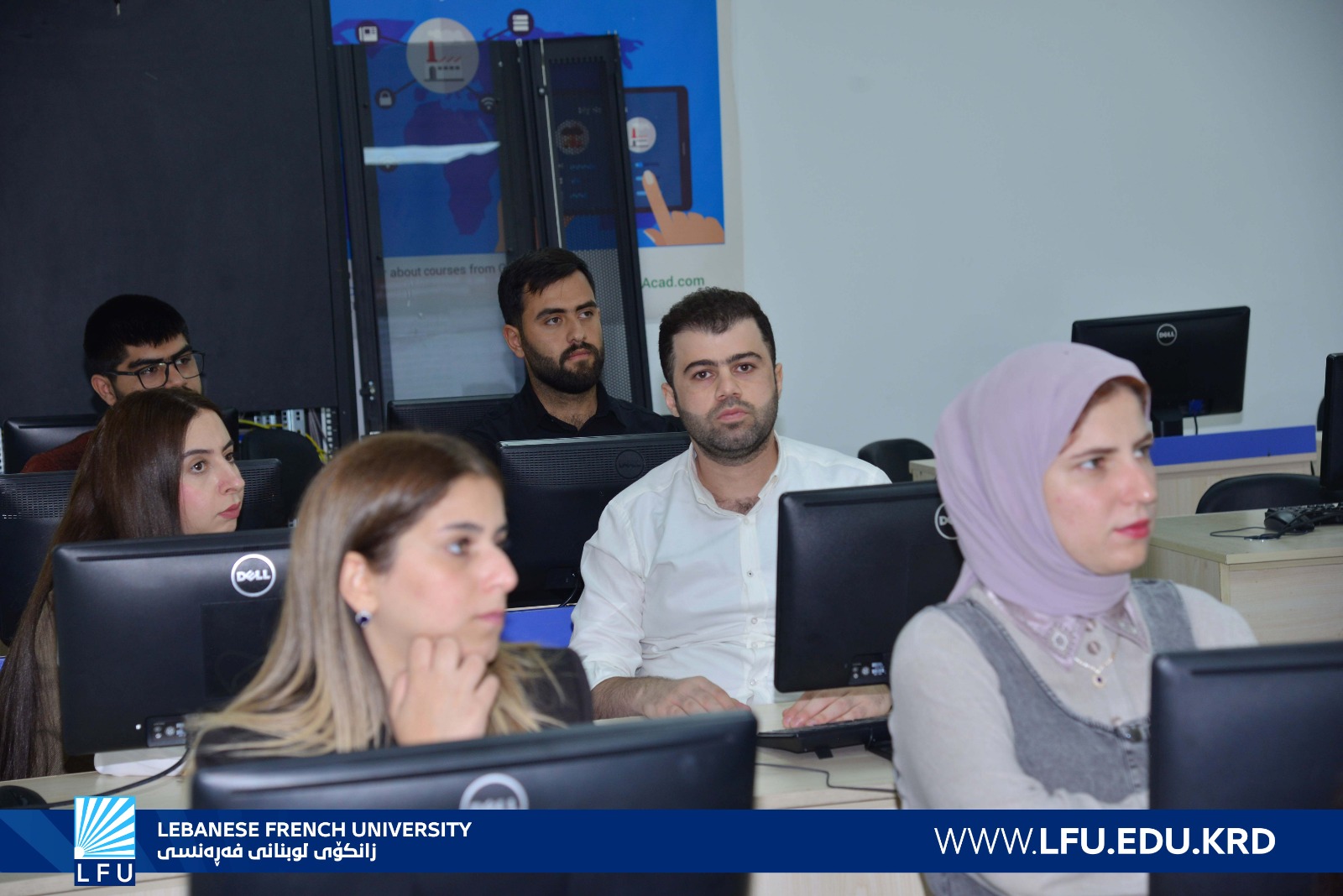
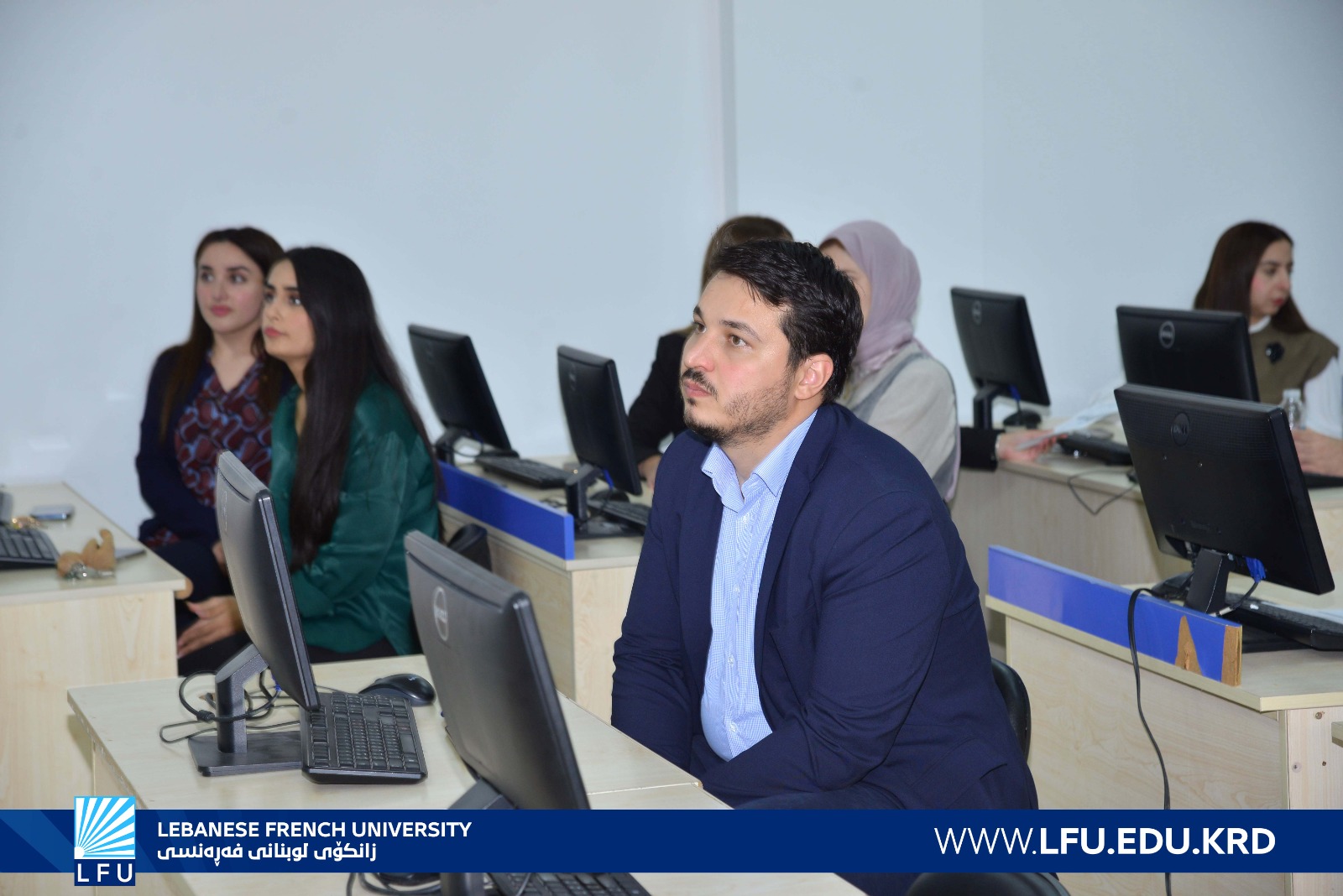
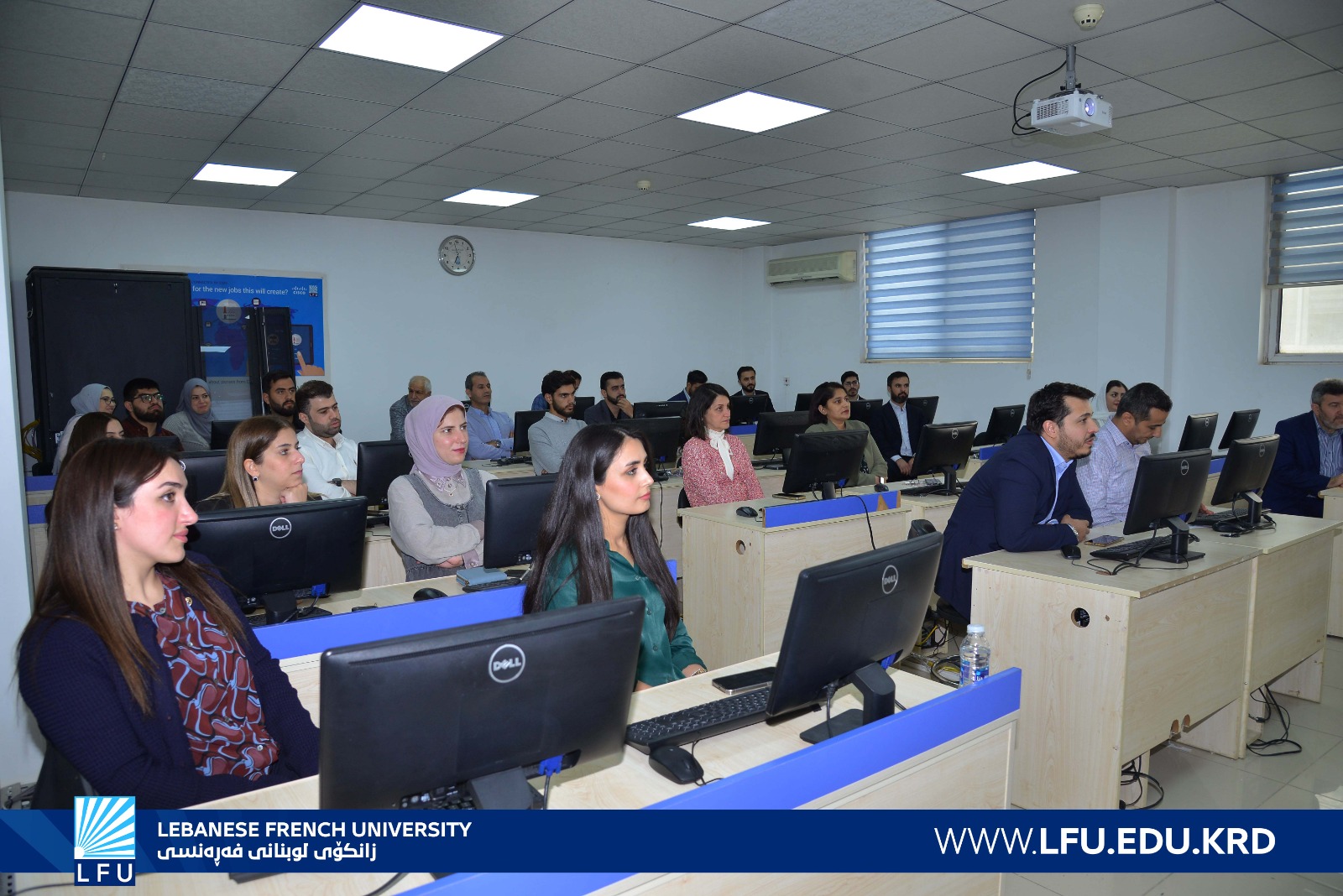
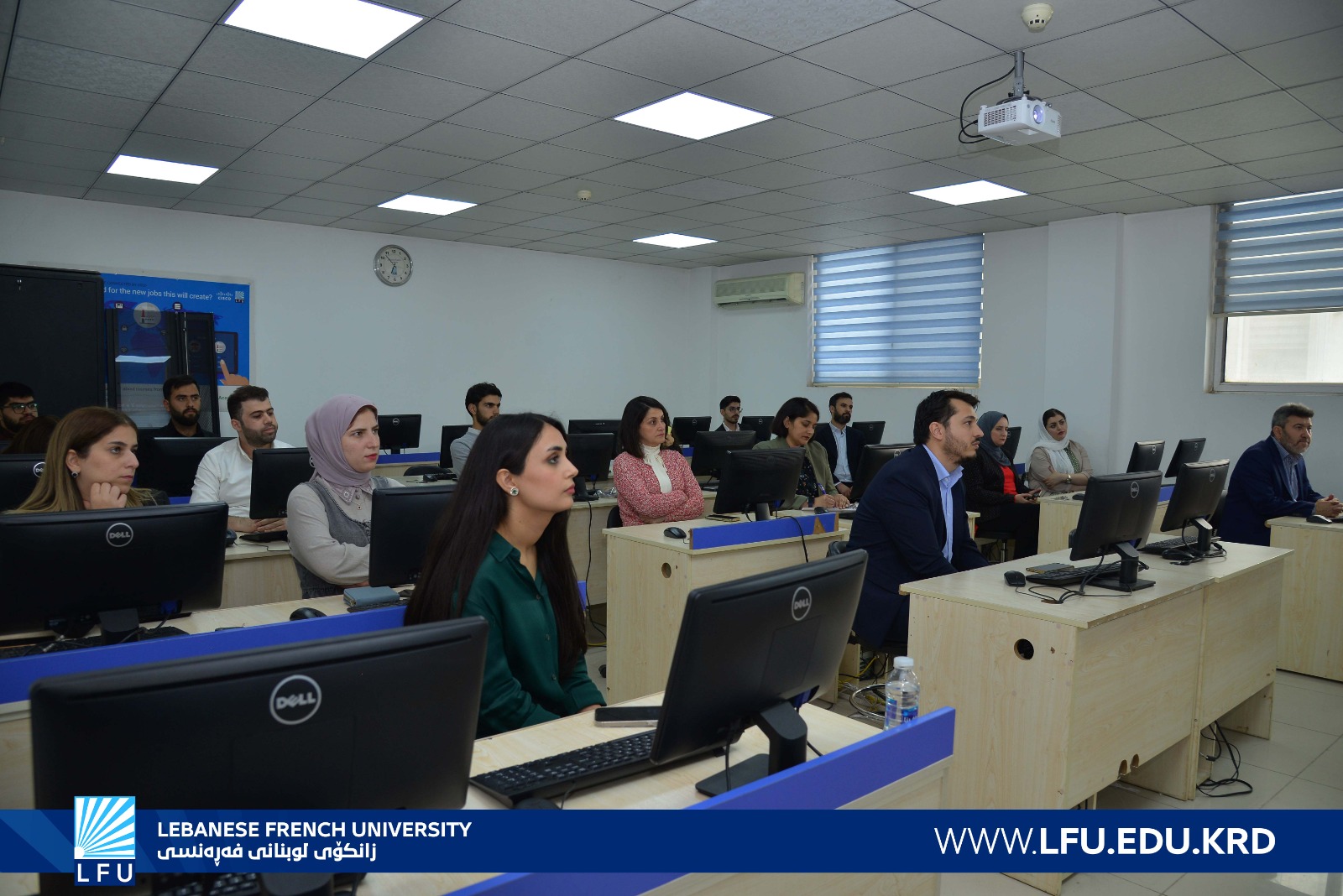