-
Data generated from social networking sites, blogs, digital magazines, and news websites is the largest human-generated data. Summarization is the process of extracting the crux of a document which when done manually can be tedious and deluging. Automatic text summarization is an approach that encapsulates long documents into a few sentences or words by enwrapping the gist and the principal information of the document. With the growth of social networking sites, eBooks, and e-Papers, the prevalence of transliterated words in text corpora is also on the rise. In this paper, we propose a word embeddings-based algorithm called HNTSumm by combining the advantages of unsupervised and supervised learning methods. The proposed algorithm HNTSumm algorithm is an imminent method for automatic text summarization of huge volumes of data that can learn word embeddings for words transliterated from other languages to English by utilizing weighted word embeddings from a Neural Embedding Model. Further, the amalgamation of extractive and abstractive approaches yields a concise and unambiguous summary of the text documents as the extractive approach eliminates redundant information. We employ a hybrid version of the Sequence-to-sequence models to generate an abstractive summary for the transliterated words. The feasibility of this algorithm was evaluated using two different news summary datasets and the accuracy scores were computed with the ROUGE evaluation metric. Experimental results corroborate the higher performance of the proposed algorithm and show HNTSumm outperforms relevant state-of-the-art algorithms for datasets with transliterated words.
- Saravana Balaji B
- International Journal of Intelligent Networks
- 10/03/2023
- https://www.sciencedirect.com/science/article/pii/S2666603023000027
- https://doi.org/10.1016/j.ijin.2023.03.001
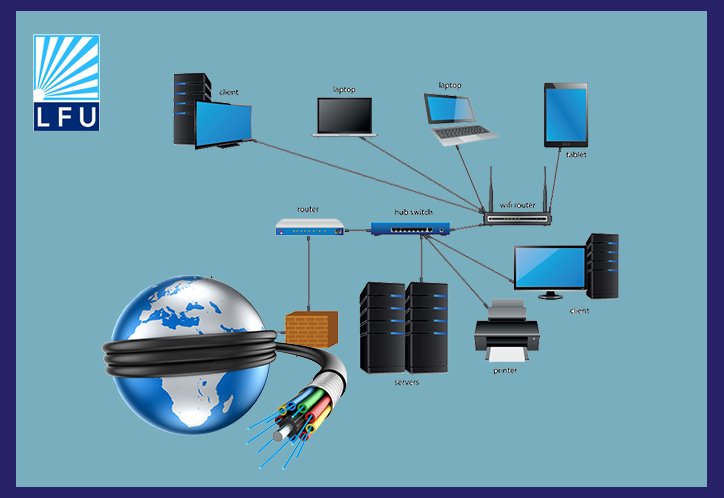