-
BACKGROUND: COVID-19 is the most dangerous virus, and its accurate diagnosis saves lives and slows its spread. However, COVID-19 diagnosis takes time and requires trained professionals. Therefore, developing a deep learning (DL) model on low-radiated imaging modalities like chest X-rays (CXRs) is needed.
OBJECTIVE: The existing DL models failed to diagnose COVID-19 and other lung diseases accurately. This study implements a multi-class CXR segmentation and classification network (MCSC-Net) to detect COVID-19 using CXR images.
METHODS: Initially, a hybrid median bilateral filter (HMBF) is applied to CXR images to reduce image noise and enhance the COVID-19 infected regions. Then, a skip connection-based residual network-50 (SC-ResNet50) is used to segment (localize) COVID-19 regions. The features from CXRs are further extracted using a robust feature neural network (RFNN). Since the initial features contain joint COVID-19, normal, pneumonia bacterial, and viral properties, the conventional methods fail to separate the class of each disease-based feature. To extract the distinct features of each class, RFNN includes a disease-specific feature separate attention mechanism (DSFSAM). Furthermore, the hunting nature of the Hybrid whale optimization
algorithm (HWOA) is used to select the best features in each class. Finally, the deep-Q-neural network (DQNN) classifies CXRs into multiple disease classes. - Gerard Deepaka, M. Madiajagan , Sanjeev Kulkarni , Ahmed Najat Ahmed , Anandbabu Gopatoti and Veeraswamy Ammisetty
- Journal of X-Ray Science and Technology
- 11 May 2023
- https://content.iospress.com/articles/journal-of-x-ray-science-and-technology/xst221360
- https://doi.org/10.3233/xst-221360
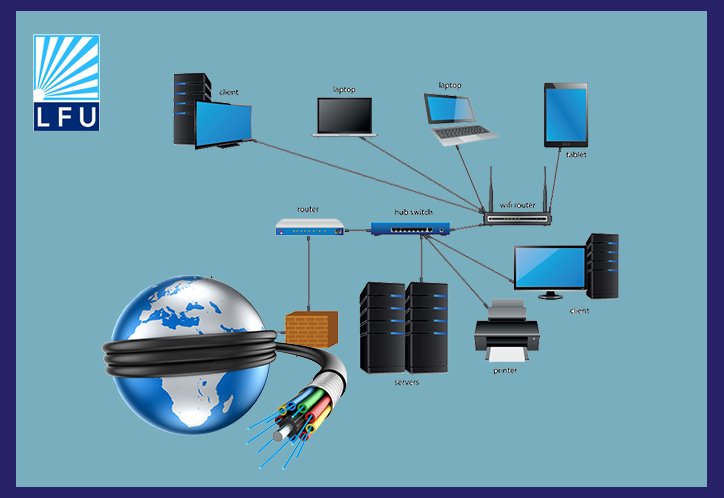