-
Smart cities are composed of intelligent industrial things that enhance people’s lives and save lives. Intelligent remote patient monitoring helps predict the patient's condition. Internet of Things (IoT), artificial intelligence (AI), and cloud computing have improved the healthcare industry. Edge computing speeds up patient data transmission and ensures latency, reliability, and response time. Nonetheless, the transmission of massive amounts of patient data may lead to IoT data security vulnerabilities, which is both a concern and a challenge. This research proposed a secure, scalable, and responsive patient monitoring system. This model used the lightweight attribute-based encryption (LABE), which encrypts and decrypts IoT patient data to protect cloud-based IoT patient data. Edge servers are positioned between the IoT and cloud to increase QoS and diagnose patient impairment. The deep belief network (DBN) predicts and monitors patient health. The bat optimization algorithm (BOA) optimizes the hyperparameters. This study used deep belief to identify hyper parameters and BOA for optimization. Swarm intelligence improves the prediction results and edge–cloud reaction time. The simulation environment assessed the secure patient health monitoring system to ensure its efficiency, security, and efficacy. The proposed model offers effective patient remote health monitoring through a secure edge–cloud–IoT environment with improved accuracy (97.9%), precision (95.6%), recall (94.6%), F1-score (94.9%), and FDR (0.06).
- Saravana Balaji B, Wiesław Paja, Milos Antonijevic, Catalin Stoean, Nebojsa Bacanin, and Miodrag Zivkovic
- Human-centric Computing and Information Sciences
- 30/04/2023
- http://hcisj.com/articles/?HCIS202313019&ckattempt=1
- https://doi.org/10.22967/HCIS.2023.13.019
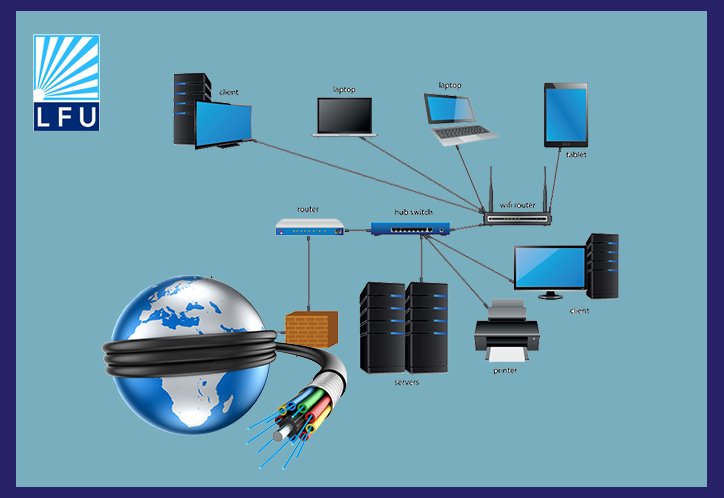